Useful predictions
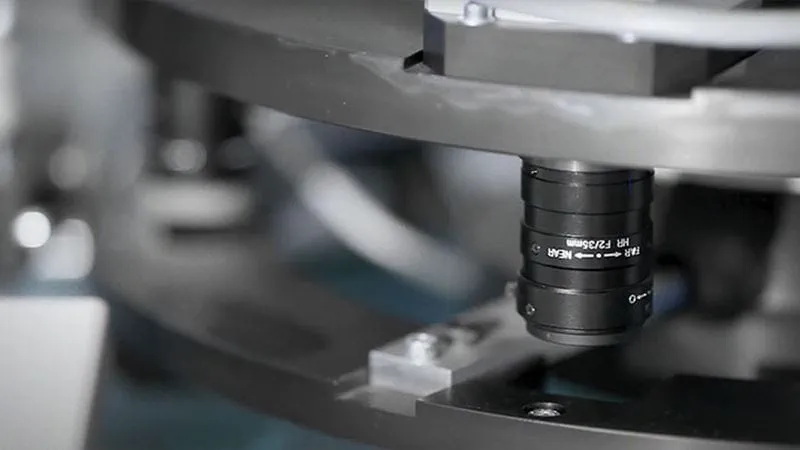
What if you could predict when a piece of equipment was going to fail? At home, few of us can cope with the downtime involved when a washing machine breaks down. At some time in its life it will, but wouldn’t it be great to know when?
Machines in production environments also break down, especially when they are in use 24/7. To manufacturers, that does not mean a heap of dirty washing, but rather the cost of lost processing time and income.
“Maintenance is an important area of focus for manufacturers,” says Dr. Johannes Kunze who is manager of digital transformation for Trelleborg Sealing Solutions. “It’s vital for process efficiency and it’s also an area where the introduction of digital technology can minimize downtime and reduce total cost of ownership, two goals at the forefront of any manufacturer’s mind.” The most basic maintenance strategy is corrective maintenance; simply fixing something when it breaks. Kunze says this is ok in noncritical applications but not a good solution if downtime is costly.
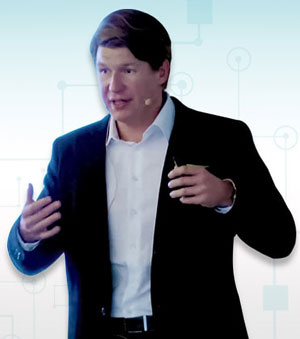
“In more critical applications, it is important that preventative maintenance is undertaken before a machine breakdown. Typically, this involves fixed planned maintenance cycles based on usage or time, but without digital technology. This can be wasteful if components are replaced before it is necessary.”
One step further is conditionbased maintenance, where sensors are added to machines to capture a prescribed condition indicating seal wear, and when a threshold is reached, that replacement is required.
Although this involves digital monitoring, it is still a stage away from full predictive maintenance, which aims to optimize maintenance time by forecasting the useful life of a component or when a component is going to fail. This is seen as one of the big value drivers of digitization and according to the McKinsey Global Institute report, The Internet of Things: Mapping the Value Beyond the Hype, it is estimated that IoT use cases such as predictive maintenance, could help companies save 630 billion USD by 2025.
Kunze adds that: “Real intelligence is achieved by making use of the sensors’ signals and predicting by means of statistics or Artificial Intelligence (AI) what is going to happen within a system and when a part is potentially going to fail, with scheduling maintenance based on this.
“For companies that are using the internet of Things (IoT) and AI in their products and its systems, putting sensors in, capturing the data and analyzing it, is not the challenge. The real challenge is to make sense of the data and act upon it.”
Good predictive maintenance depends on identifying suitable measurements to predict outages of a system.
“Talking to technicians, they will often say that they know when a system is going to fail by the noise it makes,” continues Kunze. “This is a good indicator to measure, along with other common measures, such as external temperature and vibration. However, these obvious indicators occur close to when the machine is going to fail, which is generally too late to prevent downtime.”
Sensors are increasingly used in equipment today, measuring everything from vibration, humidity, ambient temperature, to acoustic signals, motor current, insulation resistance, electrical capacitance and electrical inductance. Some even utilize thermography to create heat maps to capture what is happening inside equipment. One sensor will not generally indicate failure and data from a combination of sensors is usually required. Kunze, says: “To train a model or algorithm for digitally driven predictive maintenance, sensor data needs to be combined with good data from the field and reference data. It is necessary to find the hidden patterns, the relationship of pressures or rotational speed between breakdowns, for instance.
“One data source can be technician reports, which detail when a machine failed and what components were changed. The sensor data then needs to be split into data when the machine was operating correctly and when it was in failure mode, then integrating this engineering knowledge into preprocessing.”
It’s clear from this that predictive maintenance is not all about statistics for statistics sake. Using sensor data to create potentially meaningful indicators requires deep knowledge of specific applications and requires collaboration between engineers and data scientists to develop failure models.
“IoT will not result in the replacement of humans by robots, causing the technician or engineer to become superfluous,” confirms Kunze. “IoT requires intelligent machines, advanced analytics and critically, people.
“We’re beginning to see ever more intelligent machines, which have sensors and internet connectivity, allowing them to transmit data. People are required to make decisions, but with true predictive maintenance, these decisions are based on advanced analytics of the data that is gleaned from these intelligent machines.
“Without IoT, engineers could not possibly decipher data from up to 50 sensors, found in some of the latest manufacturing machines. Data scientists develop algorithms to find patterns and relationships within the data from these sensors and other data sources. The output from this process is more meaningful than the raw data. People then form decisions based on these actionable insights, making their work easier and significantly more effective.”
At Trelleborg Sealing Solutions, the overall approach to predictive maintenance is called Cognitive Sealing. Typically, predictive maintenance focuses on areas where there is a high cost related to downtime and maintenance. There are currently several ongoing Cognitive Sealing projects.
“One example is our three-way partnership with Vapo Hydraulics and Ampelmann Operations. This shows how big data can work to develop next generation solutions,” says Kunze. “We see this as the way we will work in the future. By helping improve our customers’ products and their customers’ products, as well as lowering their total cost of ownership, we hope to cement our relationships through our high value contribution.”
For more information, please go to:
https://www.trelleborg.com/en/seals
This is an article has been reproduced from Trelleborg's T-Time magazine. To download the latest edition, go to: www.trelleborg.com/t-time